Project
02
↳
UX/UI Design, AI, Music App
Amazon Wave AI: Deepening Your
Listening Experience
Timeframe: Jan. 2024 - Feb. 2024

Brief
In the Amazon Music Design Challenge, our team presents Wave AI, a user-friendly technology aimed at enriching how people enjoy music. Wave AI allows users to explore the layers of their favorite songs, visualize music, and personalize tracks using AI. By offering these features, we aim to enhance the connection between listeners and music, providing a more immersive and tailored listening experience.
INTRODUCTION
01
Amazon Musice Design Challenge
Our team participated this year's Amazon Music Design Challenge. Our journey starts with this prompt:
Given the current state of Amazon Music, how might AI enhance a customer’s experience with music and/or podcasts?
GENERATIVE
RESEARCH
02-1
Ways to Understand the Music Listeners
Survey: a quantitative view to understand listeners
To gain insights into music listeners' habits, interests, and preferences, we conducted a comprehensive survey aimed at understanding how individuals engage with music and access their favorite tunes. The survey was distributed across various platforms, resulting in 76 valid responses.
Interview: a qualitative view to understand listeners
We interviewed six survey participants to delve deeper into their connection with music. Through targeted questions, we explored the goals and experiences behind their music preferences and their unique listening behaviors.
GENERATIVE
RESEARCH
02-2
What We Learned: the Gap Between Music Appreciation and Active Engagement
Our findings reveal that all participants engage with music primarily through listening, with the majority accessing music via music streaming platforms.
This underscores the significant role of streaming services in making music accessible and highlights their status as the predominant avenue for music consumption in today's landscape.
In our research, two key insights emerge regarding the dynamics of music consumption and active engagement.
Insight #1: People listen to music rather than focus on it
Despite frequent music consumption, our research indicates that many listeners struggle with active engagement. While participants express a desire to focus solely on music, they often relegate it to the background while multitasking.
Insight #2: Listeners are drawn to music composition but hesitant to learn about it
Though most participants are naturally drawn to the composition of their favorite music, fewer feel that learning about it enhances their engagement. This highlights a gap between interest and effective methods for satisfying curiosity.
GENERATIVE
RESEARCH
02-3
Exploring AI Potential in Music Active Engagement
AI music track seperation
We looked at what AI can do in music industry. One popular trend is is AI track separating. Deep learning, through training on large datasets, has enabled the development of AI models capable of extracting stems such as vocals, piano, guitar, and other individual components from mixed audio tracks. Popular applications include Moises and LALAL.AI.
AI Music Search
In music exploration, AI employs advanced algorithms to match music tags in a comprehensive database, assisting users in discovering tracks based on different instruments, genre and even mood.
DEFINE
03
Problem Statement
In our research, we've noted a gap between music appreciation and active engagement. Music has become secondary to other activities.
Our problem statement is:
We aim to use AI to encourage active listening and foster a deeper appreciation of music composition for all users, regardless of their expertise.
IDEATE
04
Introduce Wave AI
We have decided to name our feature "Wave AI," merging the concept of "wave" to symbolize our focus on sound, along with "AI" to signify the integration of AI.
Features
Our new features include:
music visualizer
AI track separation
AI chord view
AI music recommendation
User flow
These enhancements are integrated into the music listening process through the following proposed user flow:
PROTOTYPE
05
Sketches & Mid-fidelity wireframes
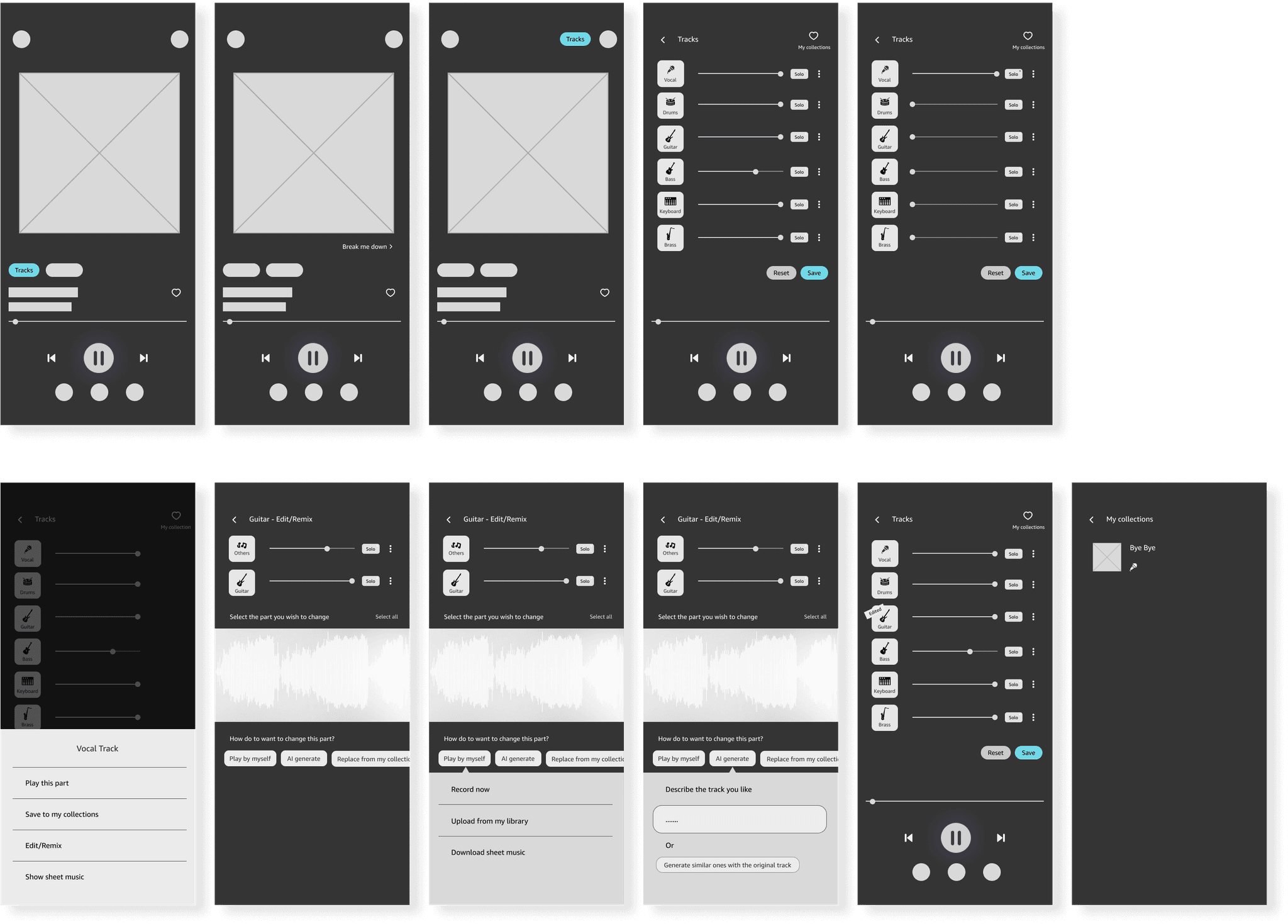
High-fidelity prototype
Here's how the high-fidelity wireframes integrate our proposed features:
Track separation flow
Start with an AI-generated visualizer displaying the music waveform.
Users can initiate the "AI Track Separation" feature by clicking a designated button.
Upon activation, the visualizer displays individual tracks for different instruments.
Users can access settings to adjust the volume levels of each instrument.
A "Solo" button allows users to isolate the sound of a specific instrument while muting others.
For users who want to know chord,
Users can view the lyrics alongside chord annotations.
This feature allows users to identify the chords being played in the song.
AI recommendation flow
AI recommendations may appear automatically or be triggered by clicking a designated banner.
Users are prompted to answer a few questions to personalize the recommendations.
Based on user input, the AI generates similar songs tailored to their preferences.
TEST
06-1
What do we want to include in user testing?
We involved the six interview participants in our user testing phase to gather qualitative feedback for refining our designs.
Through our user testing, we want to identify and discover the problem of Wave AI by users/participants.
To observe how the user’s behavior and interaction with Wave AI.
To discover what user’s experienced as opportunities for improving the design in the future.
TEST
06-2
Participants love Wave AI, but there's room for improvement
Overall, the participants think highly of our design and they are excited to use Wave AI.
They also have some common feedback for us to improve.
Feedback #1: Participants felt that the chord view was too complex.
Participants were confused by the different visualizer and the separate page.
Solution #1: Simplifying the chord view
Feedback #2: The flow for getting general AI recommendations should be more streamlined.
Participants wanted an easier way to get AI recommendations for the tracks they are interested in.
Solution #2: Streamlining AI recommendations
We've streamlined the process for accessing AI recommendations by removing the lengthy quiz. Now, users only need to indicate which tracks they'd like to explore further. Wave AI will then search for qualified songs, making the flow shorter and more user-friendly.
Feedback #3: Participants needed more clarity for getting targeted AI recommendations.
Participants were confused by selecting tracks within the visualizer to get recommendations.
Solution #3: Making AI recommendations more intuitive
We've introduced AI recommendation pop-ups directly within the song. Notable solo segments with various instruments are now tagged in the progress bar. Simply click the AI recommendation button, and Amazon Music will search for songs showcasing solos of that instrument, compiling a new playlist with similar tracks.
IMPLEMENT
07
Our Final Design
Here's our final prototype link. You can also watch the video to understand what Wave AI can do.
Our highlighted features include:
#1: AI-powered track separation & visualizer
#2: Customizable track settings
#3: Chord view
#4: Targeted AI recommendations
#5: General AI recommendations
Conclusion
08
In our project, we're not just designing for Amazon Music; we're exploring the essence of music in our lives. Though often relegated to the background, music yearns to be cherished. Through technology like AI, we aim to awaken a renewed sense of engagement, inviting users to rediscover the beauty and depth of their musical experience.